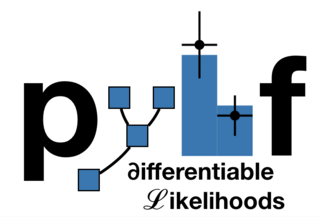
pure-python fitting/limit-setting/interval estimation HistFactory-style¶
The HistFactory p.d.f. template [CERN-OPEN-2012-016] is per-se independent of its implementation in ROOT and sometimes, it’s useful to be able to run statistical analysis outside of ROOT, RooFit, RooStats framework.
This repo is a pure-python implementation of that statistical model for multi-bin histogram-based analysis and its interval estimation is based on the asymptotic formulas of “Asymptotic formulae for likelihood-based tests of new physics” [arXiv:1007.1727]. The aim is also to support modern computational graph libraries such as PyTorch and TensorFlow in order to make use of features such as autodifferentiation and GPU acceleration.
Hello World¶
>>> import pyhf
>>> model = pyhf.simplemodels.hepdata_like(signal_data=[12.0, 11.0], bkg_data=[50.0, 52.0], bkg_uncerts=[3.0, 7.0])
>>> data = [51, 48] + model.config.auxdata
>>> test_mu = 1.0
>>> CLs_obs, CLs_exp = pyhf.infer.hypotest(test_mu, data, model, qtilde=True, return_expected=True)
>>> print(f"Observed: {CLs_obs}, Expected: {CLs_exp}")
Observed: 0.05251497423736956, Expected: 0.06445320535890459
What does it support¶
- Implemented variations:
☑ HistoSys
☑ OverallSys
☑ ShapeSys
☑ NormFactor
☑ Multiple Channels
☑ Import from XML + ROOT via uproot
☑ ShapeFactor
☑ StatError
☑ Lumi Uncertainty
- Computational Backends:
☑ NumPy
☑ PyTorch
☑ TensorFlow
☑ JAX
- Optimizers:
☑ SciPy (
scipy.optimize
)☑ MINUIT (
iminuit
)
All backends can be used in combination with all optimizers. Custom user backends and optimizers can be used as well.
Todo¶
☐ StatConfig
☐ Non-asymptotic calculators
results obtained from this package are validated against output computed from HistFactory workspaces
A one bin example¶
import pyhf
import numpy as np
import matplotlib.pyplot as plt
import pyhf.contrib.viz.brazil
pyhf.set_backend("numpy")
model = pyhf.simplemodels.hepdata_like(
signal_data=[10.0], bkg_data=[50.0], bkg_uncerts=[7.0]
)
data = [55.0] + model.config.auxdata
poi_vals = np.linspace(0, 5, 41)
results = [
pyhf.infer.hypotest(test_poi, data, model, qtilde=True, return_expected_set=True)
for test_poi in poi_vals
]
fig, ax = plt.subplots()
fig.set_size_inches(7, 5)
ax.set_xlabel(r"$\mu$ (POI)")
ax.set_ylabel(r"$\mathrm{CL}_{s}$")
pyhf.contrib.viz.brazil.plot_results(ax, poi_vals, results)
pyhf
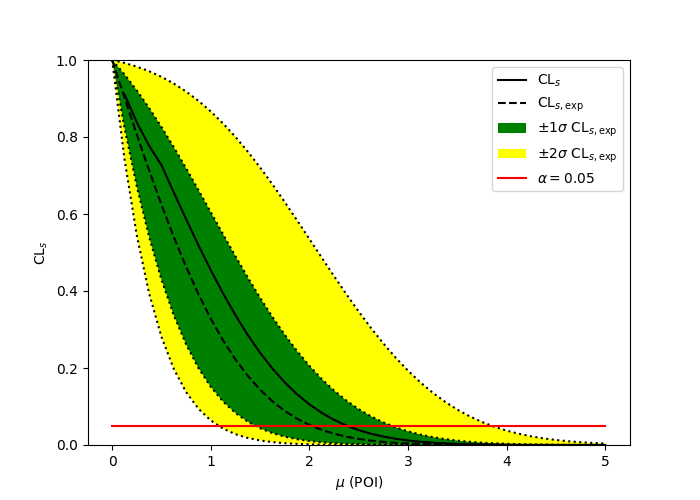
ROOT
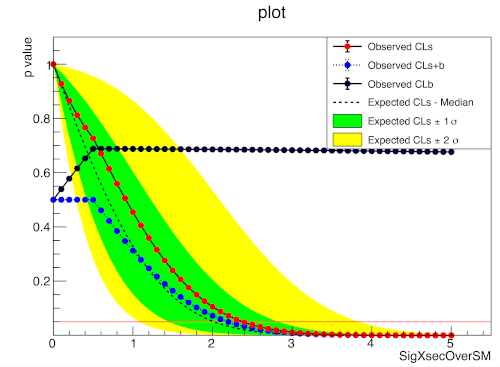
A two bin example¶
import pyhf
import numpy as np
import matplotlib.pyplot as plt
import pyhf.contrib.viz.brazil
pyhf.set_backend("numpy")
model = pyhf.simplemodels.hepdata_like(
signal_data=[30.0, 45.0], bkg_data=[100.0, 150.0], bkg_uncerts=[15.0, 20.0]
)
data = [100.0, 145.0] + model.config.auxdata
poi_vals = np.linspace(0, 5, 41)
results = [
pyhf.infer.hypotest(test_poi, data, model, qtilde=True, return_expected_set=True)
for test_poi in poi_vals
]
fig, ax = plt.subplots()
fig.set_size_inches(7, 5)
ax.set_xlabel(r"$\mu$ (POI)")
ax.set_ylabel(r"$\mathrm{CL}_{s}$")
pyhf.contrib.viz.brazil.plot_results(ax, poi_vals, results)
pyhf
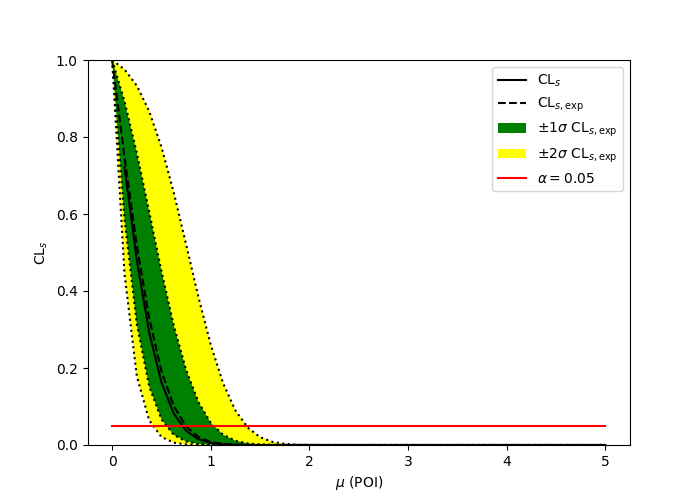
ROOT
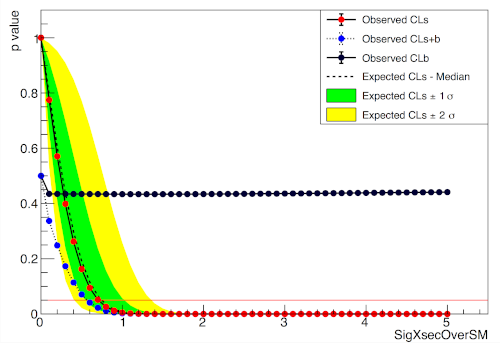
Installation¶
To install pyhf
from PyPI with the NumPy backend run
python -m pip install pyhf
and to install pyhf
with all additional backends run
python -m pip install pyhf[backends]
or a subset of the options.
To uninstall run
python -m pip uninstall pyhf
Questions¶
If you have a question about the use of pyhf
not covered in the
documentation, please ask a question
on Stack Overflow
with the [pyhf]
tag, which the pyhf
dev team
watches.
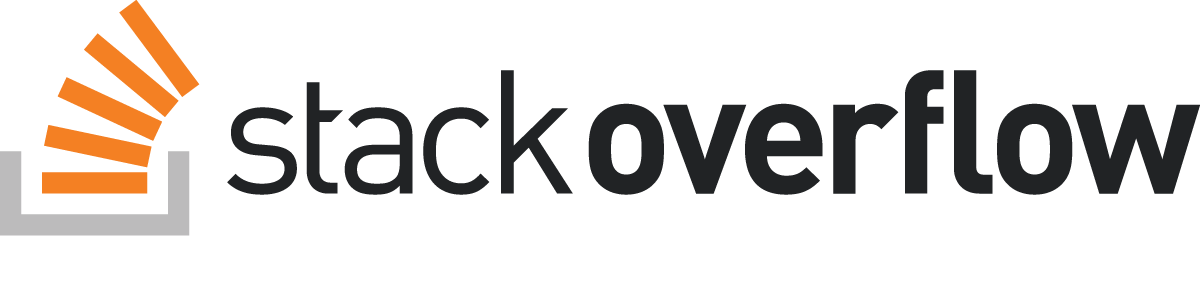
If you believe you have found a bug in pyhf
, please report it in the
GitHub
Issues.
Citation¶
As noted in Use and
Citations, the preferred
BibTeX entry for citation of pyhf
is
@software{pyhf,
author = "{Heinrich, Lukas and Feickert, Matthew and Stark, Giordon}",
title = "{pyhf: v0.5.2}",
version = {0.5.2},
doi = {10.5281/zenodo.1169739},
url = {https://github.com/scikit-hep/pyhf},
}
Authors¶
pyhf
is openly developed by Lukas Heinrich, Matthew Feickert, and Giordon Stark.
Please check the contribution statistics for a list of contributors.
Acknowledgements¶
Matthew Feickert has received support to work on pyhf
provided by NSF
cooperative agreement OAC-1836650 (IRIS-HEP)
and grant OAC-1450377 (DIANA/HEP).